Universal Bovine Identification Asheesh Sharma
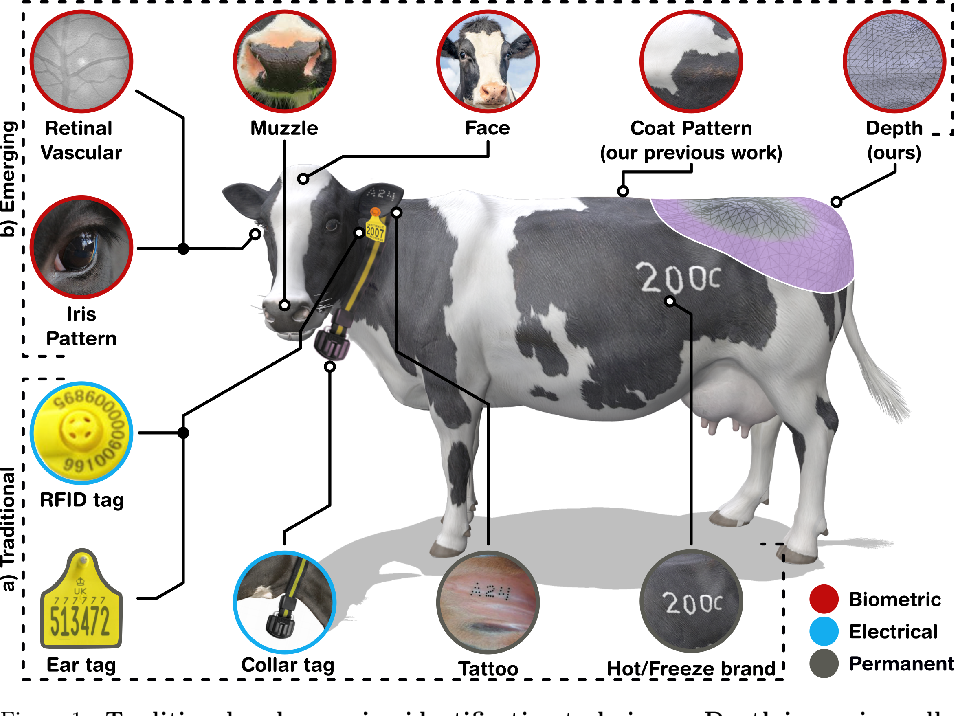
Figure 1 From Universal Bovine Identification Via Depth Data And Deep View a pdf of the paper titled universal bovine identification via depth data and deep metric learning, by asheesh sharma and 9 other authors. this paper proposes and evaluates, for the first time, a top down (dorsal view), depth only deep learning system for accurately identifying individual cattle and provides associated code, datasets, and. This talk was part of the ‘ai in infectious diseases’ workshop held on 18 april 2024, hosted by elizabeth blackwell institute and the infection and immunity.
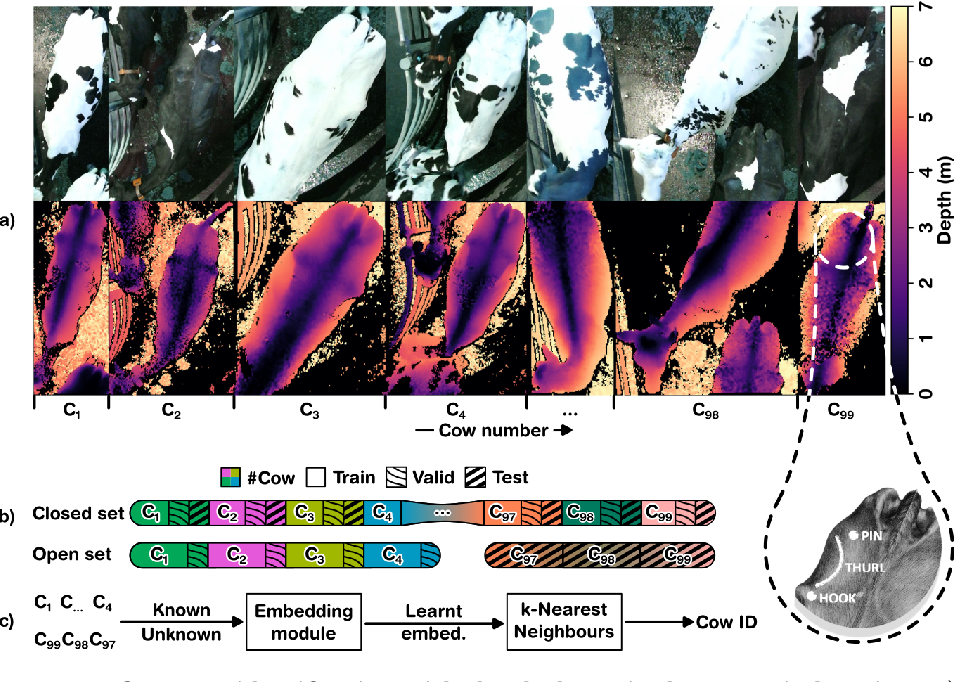
Figure 2 From Universal Bovine Identification Via Depth Data And Deep Universal bovine identification via depth data and deep metric learning. asheesh sharma, lucy randewich, william andrew, sion hannuna, neill campbell, siobhan mullan, andrew w. dowsey*, melvyn smith *, mark hansen, tilo burghardt. *corresponding author for this work. bristol veterinary school. bristol doctoral college. school of computer science. Furthermore, this study opens the door for the individual identification of cattle breeds without coat patterns: 98% of beef, 49% of dairy and 17% dual purpose cattle in the u.k. (see p. 18, [7]). figure 2: open set identification with depth data via deep metric learning. a) examples of top down rgb depth images in our dataset, pairs correspond. Doi: 10.48550 arxiv.2404.00172 corpus id: 268819946; universal bovine identification via depth data and deep metric learning @article{sharma2024universalbi, title={universal bovine identification via depth data and deep metric learning}, author={asheesh sharma and lucy randewich and william andrew and sion l. hannuna and neill campbell and siobhan mullan and andrew w dowsey and melvyn smith. B) as the value of n is increased from 2 to 10, more images are discarded from the train set, resulting in some cows with no images left. these cows in the test set are considered ‘unknowns’, i.e. part of the open set, enrolled with k nn, post training. "universal bovine identification via depth data and deep metric learning".
Comments are closed.