Understanding Few Shot Multi Task Representation Learning Theory в The
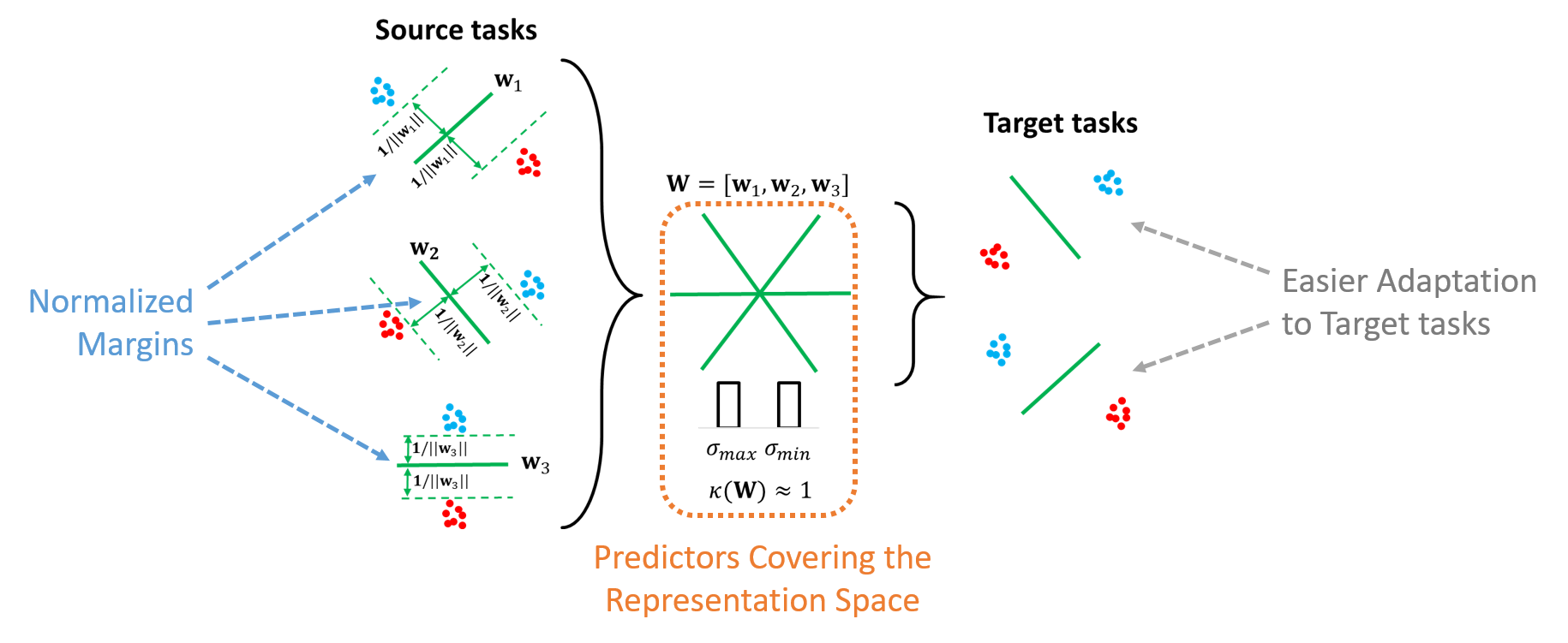
Understanding Few Shot Multi Task Representation Learning Multi task representation learning (mtr) is a popular paradigm to learn shared representations from multiple related tasks. it has demonstrated its efficiency for solving different problems, ranging from machine translation for natural language processing to object detection in computer vision. on the other hand, few shot learning is a recent. The main idea of multi task representation learning is that all the tasks considered are related by an underlying common representation, where the latter is learned by jointly training on these tasks. in the seminal work on multi task representation learning theory, baxter 2 defined what they call environments of tasks. this means that the.
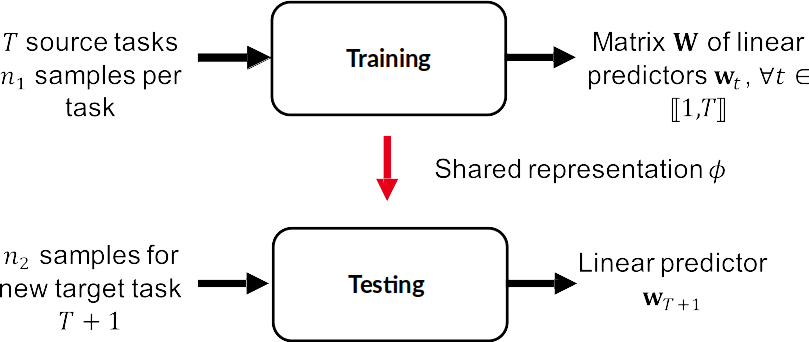
Understanding Few Shot Multi Task Representation Learning In this post, we dive into a recent iclr 2021 paper by du et al. that demonstrated novel learning bounds for multi task learning in the few shot setting and go beyond it by establishing the connections that allow to better understand the inner workings of meta learning algorithms as well. pdf abstract. In this paper, we consider the framework of multi task representation (mtr) learning where the goal is to use source tasks to learn a representation that reduces the sample complexity of solving a target task. we start by reviewing recent advances in mtr theory and show that they can provide novel insights for popular meta learning algorithms when analyzed within this framework. in particular. Improving few shot learning through multi task representation learning theory quentinbouniot1, 2[0000 −00020982 372x],ievgenredko 3860 5502], romaricaudigier 1[0000 −0002 4757 2052],angéliqueloesch 0001 5427 3010], amauryhabrard2,3[0000 −0003 3038 9347] 1 universitéparis saclay,cea,list,f 91120,palaiseau,france {firstname.lastname}@cea.fr. Abstract. in this paper, we consider the framework of multi task representation (mtr) learning where the goal is to use source tasks to learn a representation that reduces the sample complexity of.
Comments are closed.