Statistics Lecture 8 2 Part 8

Statistics Lecture 8 2 Part 8 Youtube Patreon professorleonardstatistics lecture 8.2: an introduction to hypothesis testing. This page titled 8.1.2: introduction to hypothesis testing part 2 is shared under a cc by 4.0 license and was authored, remixed, and or curated by openstax via source content that was edited to the style and standards of the libretexts platform. in every hypothesis test, the outcomes are dependent on a correct interpretation of the data.
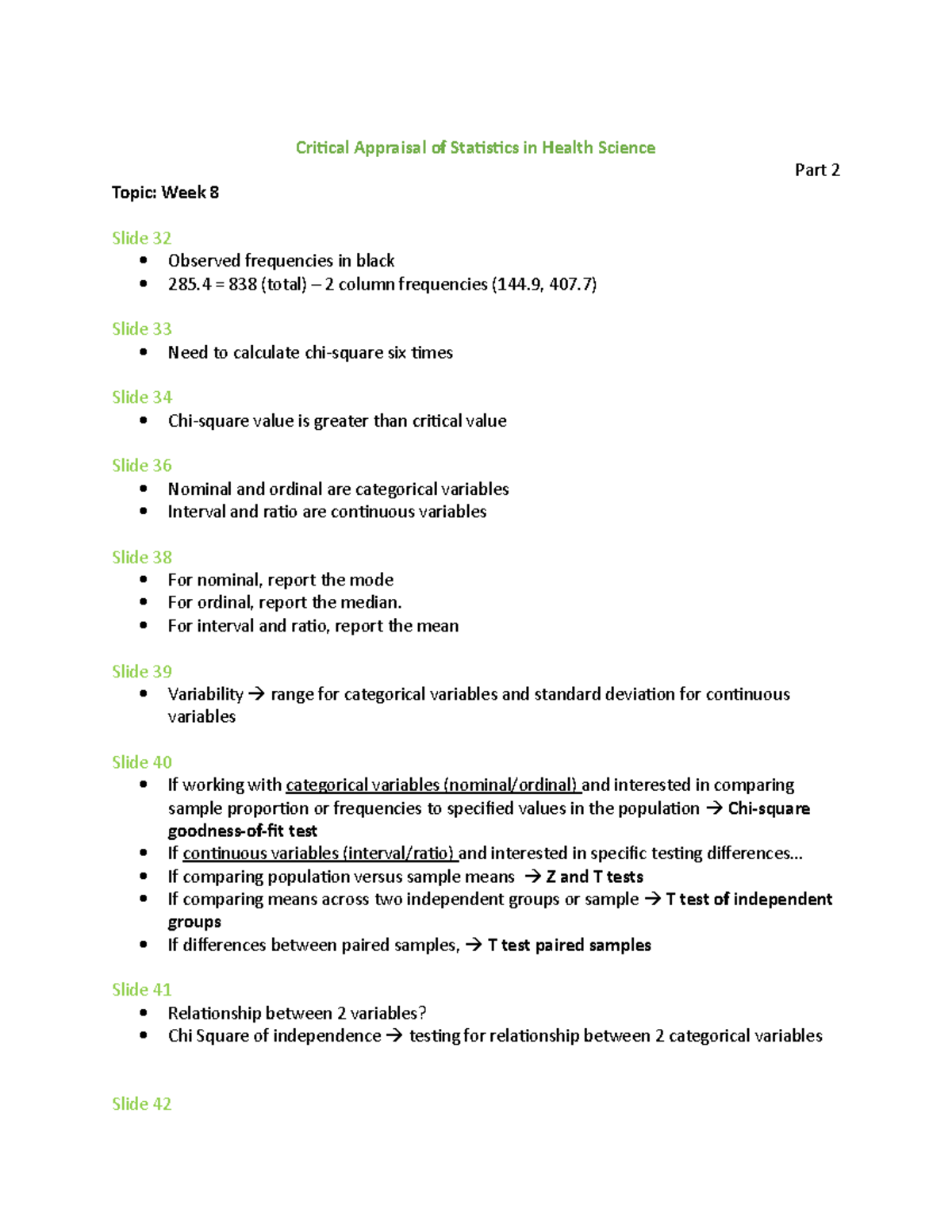
Critical Appraisal Of Statistics In Health Science Lecture 8 Part 2 Patreon professorleonardstatistics lecture 8.3: hypothesis testing for population proportion. testing a claim about a population proportion. Statistical tests signal & background. the probability to reject a background hypothesis for background events is called the background efficiency: 1. b = g(t; b)dt = ↵. tcut. the probability to accept a signal event as signal is the signal efficiency: s = z g(t; s)dt = 1. tcut. Empirical risk minimization. 1. pattern classification: θ : x → {0, 1}, z = (x, y) ∈ x × {0, 1}, l(θ, (x, y)) = 1[θ(x) 6= y]. empirical risk minimization chooses θ to minimize misclassifications on the sample. 2. density estimation: p is a density, x. erm is maximum likelihood. ∼ p , p , l(θ, z) = − log θ∗ pθ(z). The area to the left of the critical value \(z {\alpha 2}\) and to the right of the critical value \(z {1 \alpha 2}\) is called the critical or rejection region. see figure 8 9. figure 8 9. when \(\alpha\) = 0.05 then the critical values \(z {\alpha 2}\) and \(z {1 \alpha 2}\) are found using the following technology.

Lecture 8 2 Docx 1 Lecture 11 11 3 22 Chapter 8 Statistics Secti Empirical risk minimization. 1. pattern classification: θ : x → {0, 1}, z = (x, y) ∈ x × {0, 1}, l(θ, (x, y)) = 1[θ(x) 6= y]. empirical risk minimization chooses θ to minimize misclassifications on the sample. 2. density estimation: p is a density, x. erm is maximum likelihood. ∼ p , p , l(θ, z) = − log θ∗ pθ(z). The area to the left of the critical value \(z {\alpha 2}\) and to the right of the critical value \(z {1 \alpha 2}\) is called the critical or rejection region. see figure 8 9. figure 8 9. when \(\alpha\) = 0.05 then the critical values \(z {\alpha 2}\) and \(z {1 \alpha 2}\) are found using the following technology. This unit's exercises do not count toward course mastery. unit 14 unit 14: inference for categorical data (chi square tests) test statistic and p value in a goodness of fit test. expected counts in chi squared tests with two way tables. test statistic and p value in chi square tests with two way tables. 8 4 lecture 8: nonparametric regression 8.3 theory now we study some statistical properties of the estimator mb h. we skip the details of derivations4. bias. the bias of the kernel regression at a point xis bias(mb h(x)) = h2 2 k m00(x) 2 m0(x)p0(x) p(x) o(h2); where p(x) is the probability density function of the covariates x 1; 2;x n and.

Example Of Lecture 8 2 Statistics Youtube This unit's exercises do not count toward course mastery. unit 14 unit 14: inference for categorical data (chi square tests) test statistic and p value in a goodness of fit test. expected counts in chi squared tests with two way tables. test statistic and p value in chi square tests with two way tables. 8 4 lecture 8: nonparametric regression 8.3 theory now we study some statistical properties of the estimator mb h. we skip the details of derivations4. bias. the bias of the kernel regression at a point xis bias(mb h(x)) = h2 2 k m00(x) 2 m0(x)p0(x) p(x) o(h2); where p(x) is the probability density function of the covariates x 1; 2;x n and.
Comments are closed.