Introduction To Multi Task Prompt Tuning Niklas Heidloff
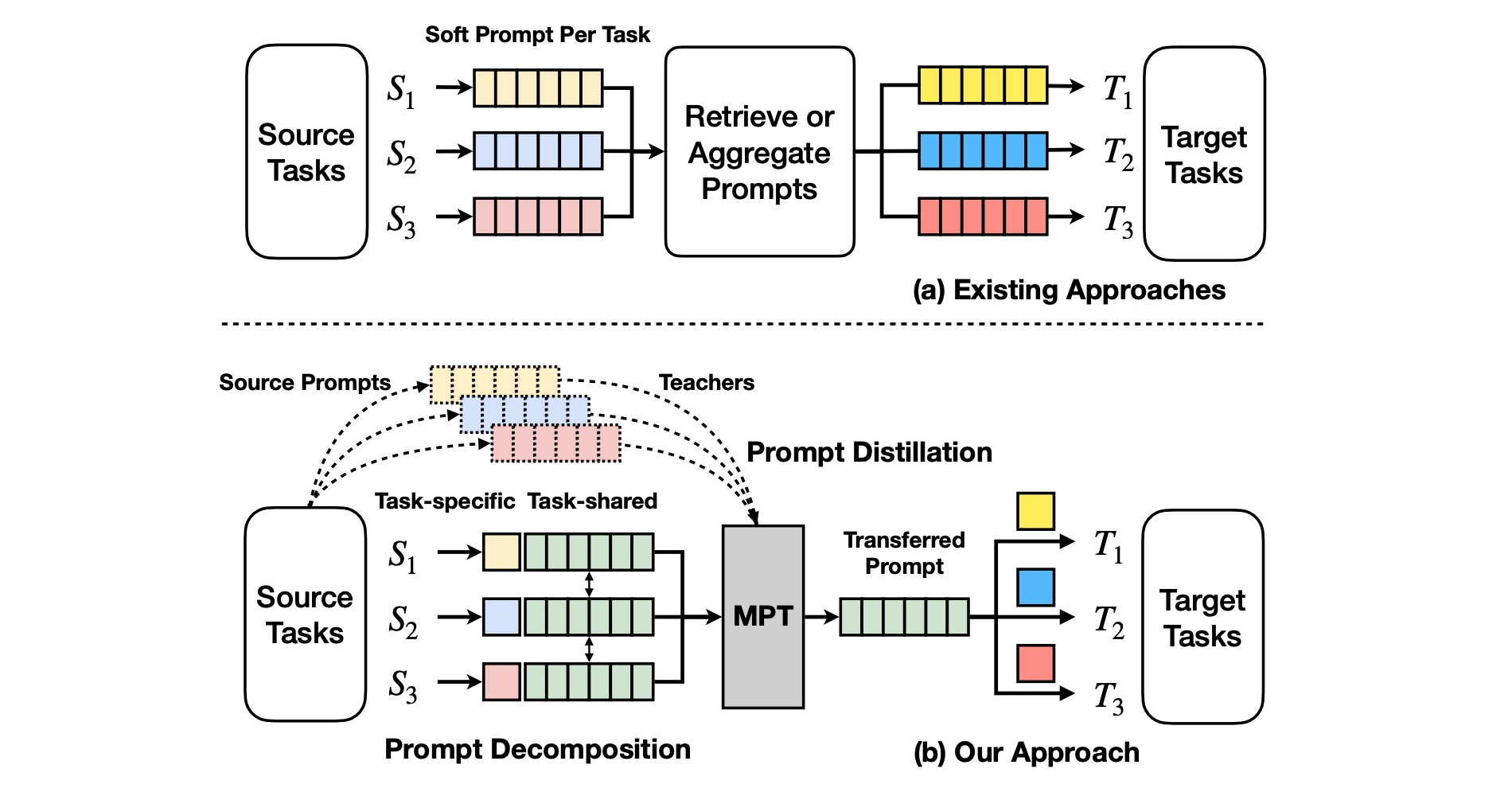
Introduction To Multi Task Prompt Tuning Niklas Heidloff Multi task prompt tuning is an evolution of prompt tuning. the graphic at the top of this post describes the main idea. instead of retrieving or aggregating source prompts (top), multitask prompt tuning (mpt, bottom) learns a single transferable prompt. the transferable prompt is learned via prompt decomposition and distillation. Introduction to prompt tuning. posted mar 2, 2023. by niklas heidloff. 4 min read. training foundation models and even fine tuning models for custom domains is expensive and requires lots of resources. to avoid changing the pretrained models, a new more resource efficient technique has emerged, called prompt tuning.
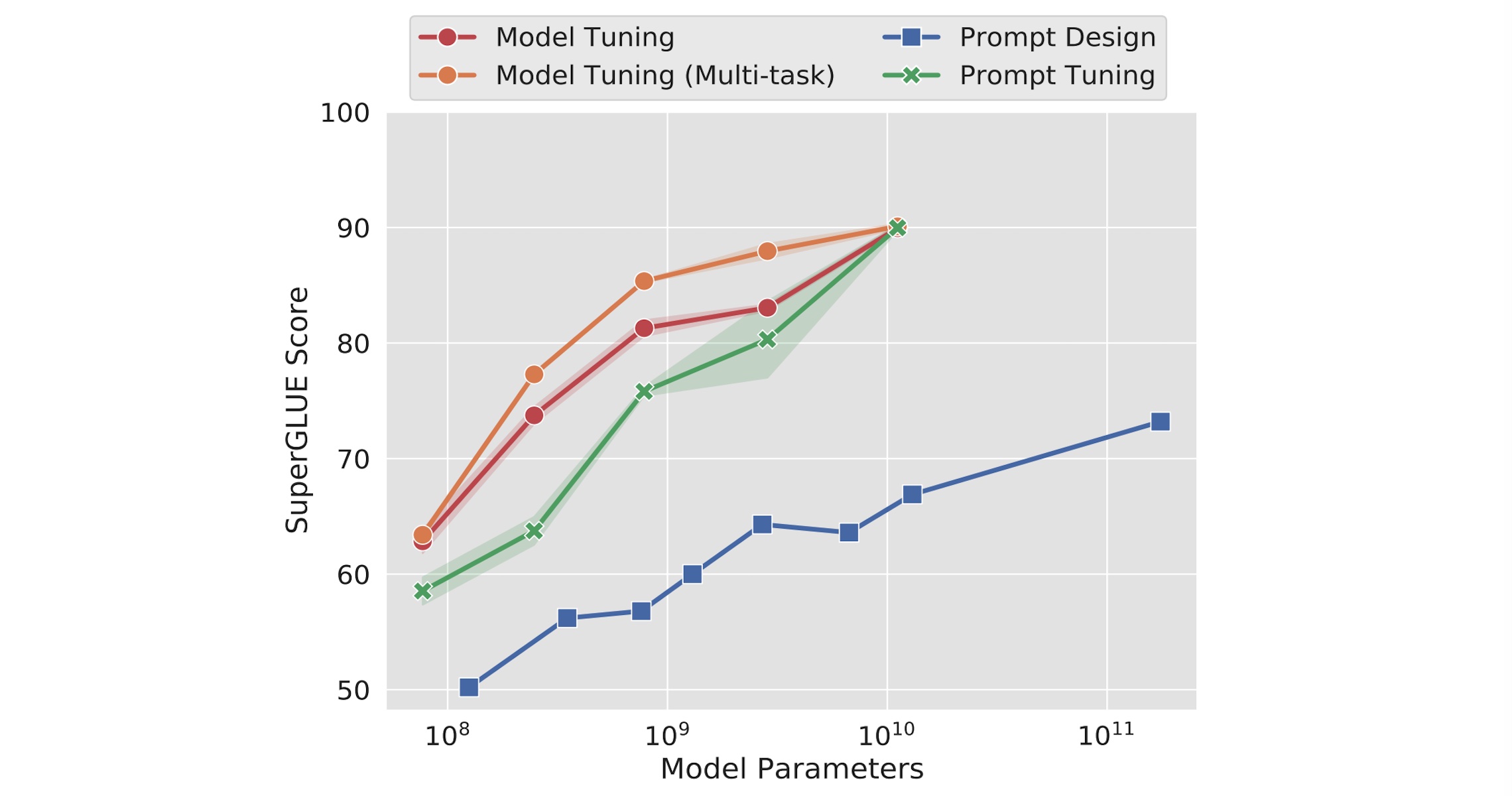
Introduction To Multi Task Prompt Tuning Niklas Heidloff 07 mar introduction to multi task prompt tuning; 06 mar the importance of prompt engineering; 04 mar running the large language model flan t5 locally; 03 mar introduction to prompt tuning; 28 feb chatgpt like functionality in ibm watson assistant; 24 feb foundation models, transformers, bert and gpt; 16 feb ibm watson nlp use cases. We propose multitask prompt tuning (mpt), which first learns a single transferable prompt by distilling knowledge from multiple task specific source prompts. we then learn multiplicative low rank updates to this shared prompt to efficiently adapt it to each downstream target task. extensive experiments on 23 nlp datasets demonstrate that our. On my blog: introduction to multi task prompt tuning #ai #llm #foundationmodels #ibmresearch #innovation lnkd.in ebeeki6g. View a pdf of the paper titled multitask prompt tuning enables parameter efficient transfer learning, by zhen wang and 5 other authors. prompt tuning, in which a base pretrained model is adapted to each task via conditioning on learned prompt vectors, has emerged as a promising approach for efficiently adapting large language models to multiple.
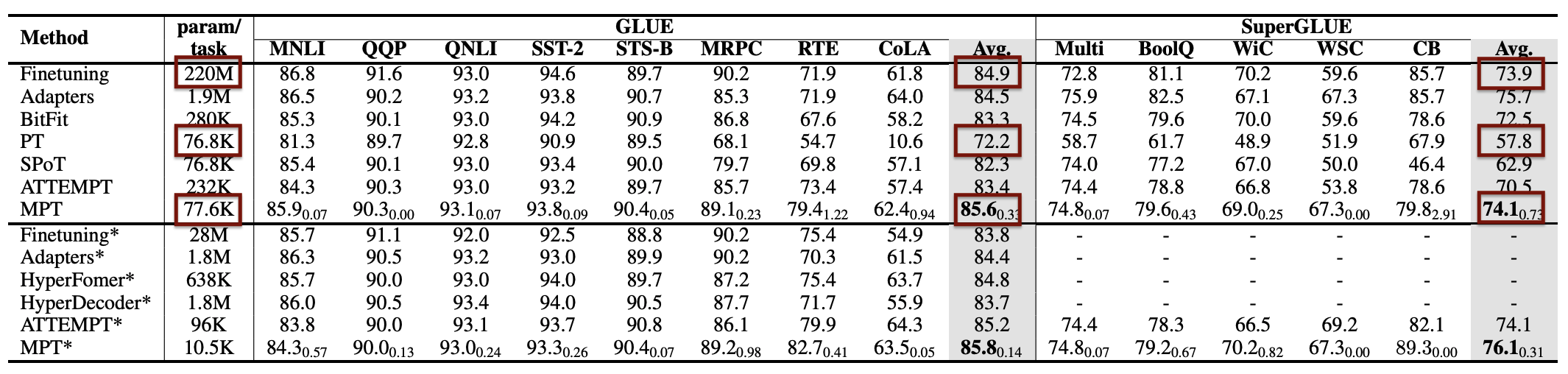
Introduction To Multi Task Prompt Tuning Niklas Heidloff On my blog: introduction to multi task prompt tuning #ai #llm #foundationmodels #ibmresearch #innovation lnkd.in ebeeki6g. View a pdf of the paper titled multitask prompt tuning enables parameter efficient transfer learning, by zhen wang and 5 other authors. prompt tuning, in which a base pretrained model is adapted to each task via conditioning on learned prompt vectors, has emerged as a promising approach for efficiently adapting large language models to multiple. In recent years, multi task prompt tuning has garnered considerable attention for its inherent modularity and potential to enhance parameter efficient transfer learning across diverse tasks. this paper aims to analyze and improve the performance of multiple tasks by facilitating the transfer of knowledge between their corresponding prompts in a multi task setting. our proposed approach. To this end, we propose multitask vision language prompt tuning (mvlpt), to the best of our knowledge, the first method incorporating the cross task knowledge into vision language prompt tuning. mvlpt is a simple yet effec tive way to enable information sharing between multiple tasks. mvlpt consists of two stages: multitask source prompt.
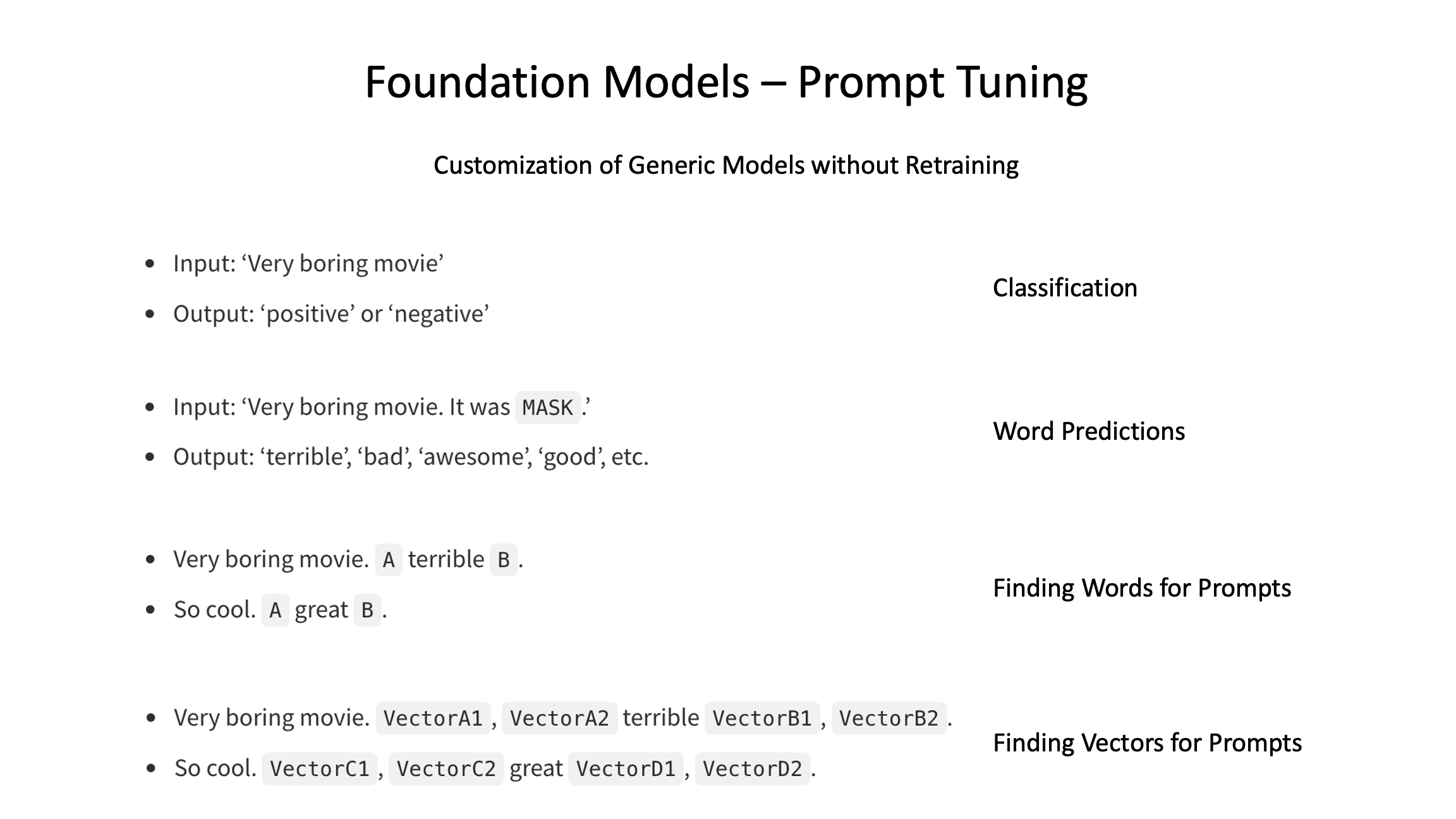
Introduction To Prompt Tuning Niklas Heidloff In recent years, multi task prompt tuning has garnered considerable attention for its inherent modularity and potential to enhance parameter efficient transfer learning across diverse tasks. this paper aims to analyze and improve the performance of multiple tasks by facilitating the transfer of knowledge between their corresponding prompts in a multi task setting. our proposed approach. To this end, we propose multitask vision language prompt tuning (mvlpt), to the best of our knowledge, the first method incorporating the cross task knowledge into vision language prompt tuning. mvlpt is a simple yet effec tive way to enable information sharing between multiple tasks. mvlpt consists of two stages: multitask source prompt.
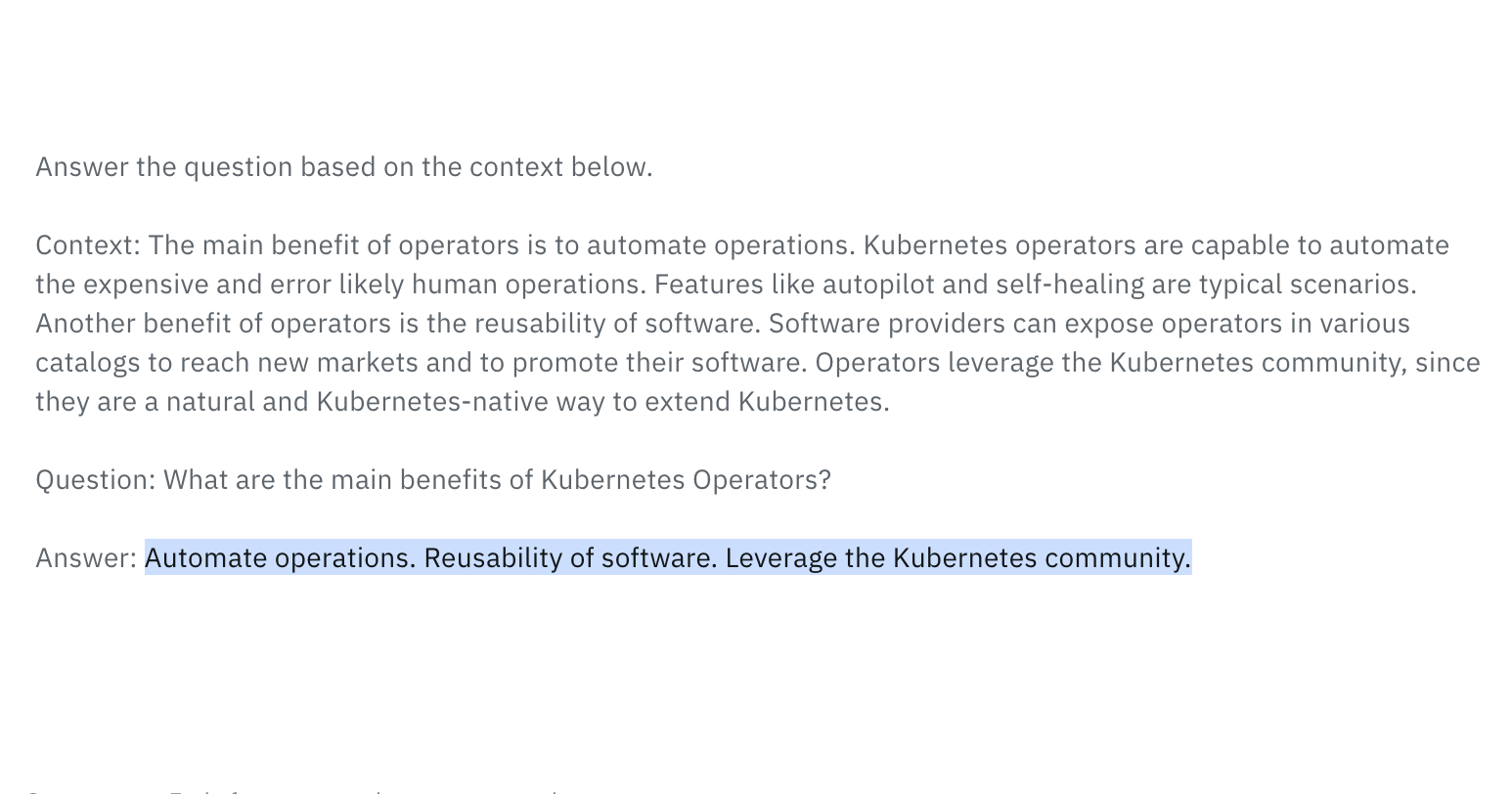
The Importance Of Prompt Engineering Niklas Heidloff
Comments are closed.