Figure 2 From Universal Bovine Identification Via Depth Data And Deep
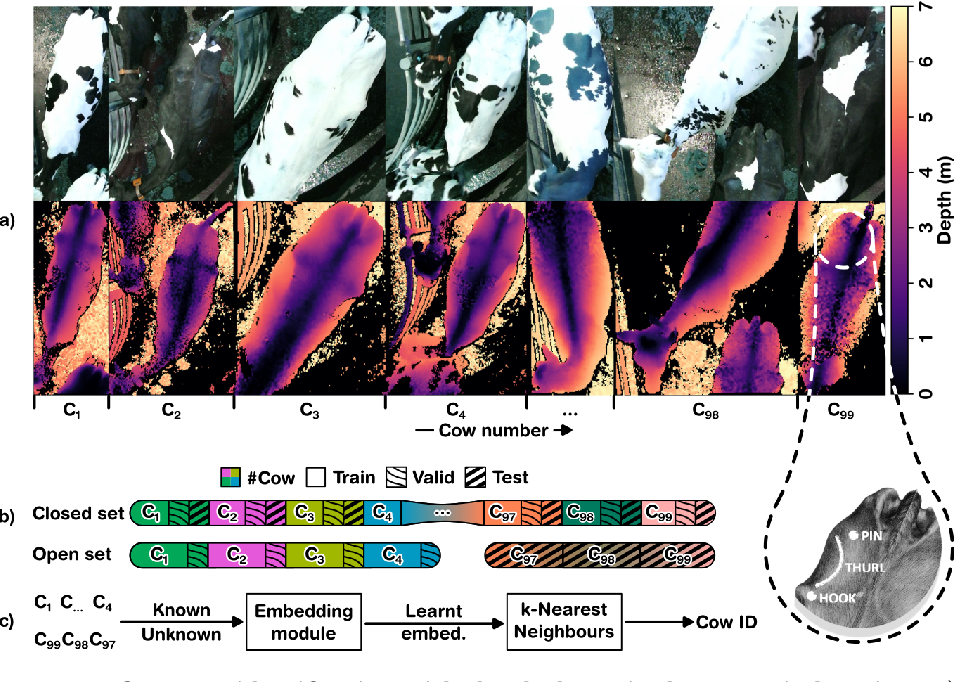
Figure 2 From Universal Bovine Identification Via Depth Data And Deep View a pdf of the paper titled universal bovine identification via depth data and deep metric learning, by asheesh sharma and 9 other authors. this paper proposes and evaluates, for the first time, a top down (dorsal view), depth only deep learning system for accurately identifying individual cattle and provides associated code, datasets, and. Furthermore, this study opens the door for the individual identification of cattle breeds without coat patterns: 98% of beef, 49% of dairy and 17% dual purpose cattle in the u.k. (see p. 18, [7]). figure 2: open set identification with depth data via deep metric learning. a) examples of top down rgb depth images in our dataset, pairs correspond.
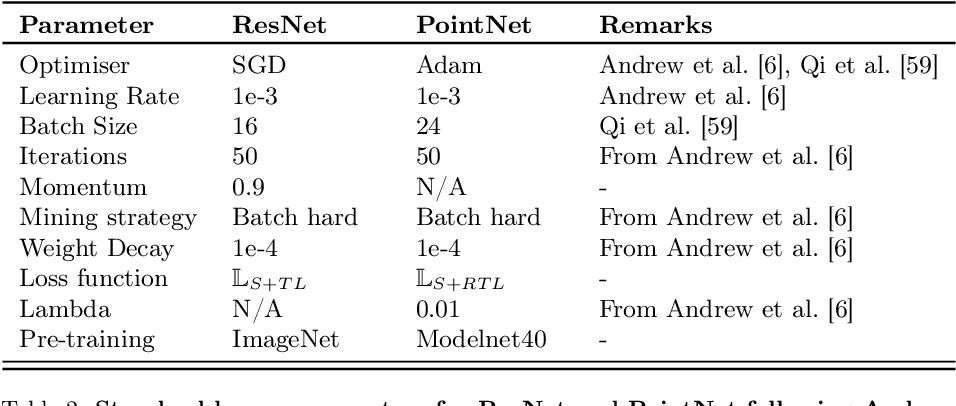
Table 2 From Universal Bovine Identification Via Depth Dataођ A deep metric learning method for cattle identification using depth data from an off the shelf 3d camera that relies on cnn and mlp backbones that learn well generalised embedding spaces from the body shape to differentiate individuals, thus eliminating the need to retrain the network for enrolling new individuals. this paper proposes and evaluates, for the first time, a top down (dorsal view. Underpinned by our previous work, this paper introduces a deep metric learning method for cattle identification using depth data from an off the shelf 3d camera. the method relies on cnn and mlp backbones that learn well generalised embedding spaces from the body shape to differentiate individuals requiring neither species specific coat. Figure 6: network architectures a) the resnet 50 and pointnet architectures consume depth maps and point clouds as inputs, respectively. the resnet network consists of 4 convolution blocks; the double arrows between the blocks represent the skip connections he et al. [65]. b) the output from the last convolution block is fed to the spatial context module (scm), followed by the average pooling. Universal bovine identification via depth data and deep metric learning: paper and code. this paper proposes and evaluates, for the first time, a top down (dorsal view), depth only deep learning system for accurately identifying individual cattle and provides associated code, datasets, and training weights for immediate reproducibility. an increase in herd size skews the cow to human ratio at.
Comments are closed.