Brain Encoding And Decoding In Fmri With Bidirectional Deep Generative
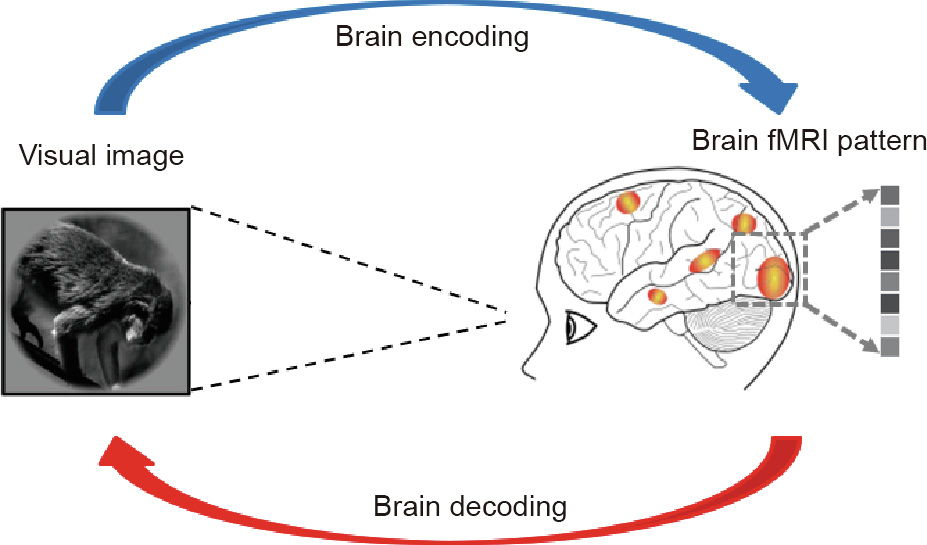
Brain Encoding And Decoding In Fmri With Bidirectional Deep Generative Fig. 1. brain encoding and decoding in fmri. the encoding model attempts to predict brain responses based on the presented visual stimuli, while the decoding model attempts to infer the corresponding visual stimuli by analyzing the observed brain responses. in practice, encoding and decoding models should not be seen as mutually exclusive. 3. brain decoding with deep generative models a promising research direction involves the integration of deep learning methods into brain decoding research. deep generative models such as variational autoencoders (vaes) [45,46] and generative adversarial networks (gans) [47] have achieved great success in the field of image generation.
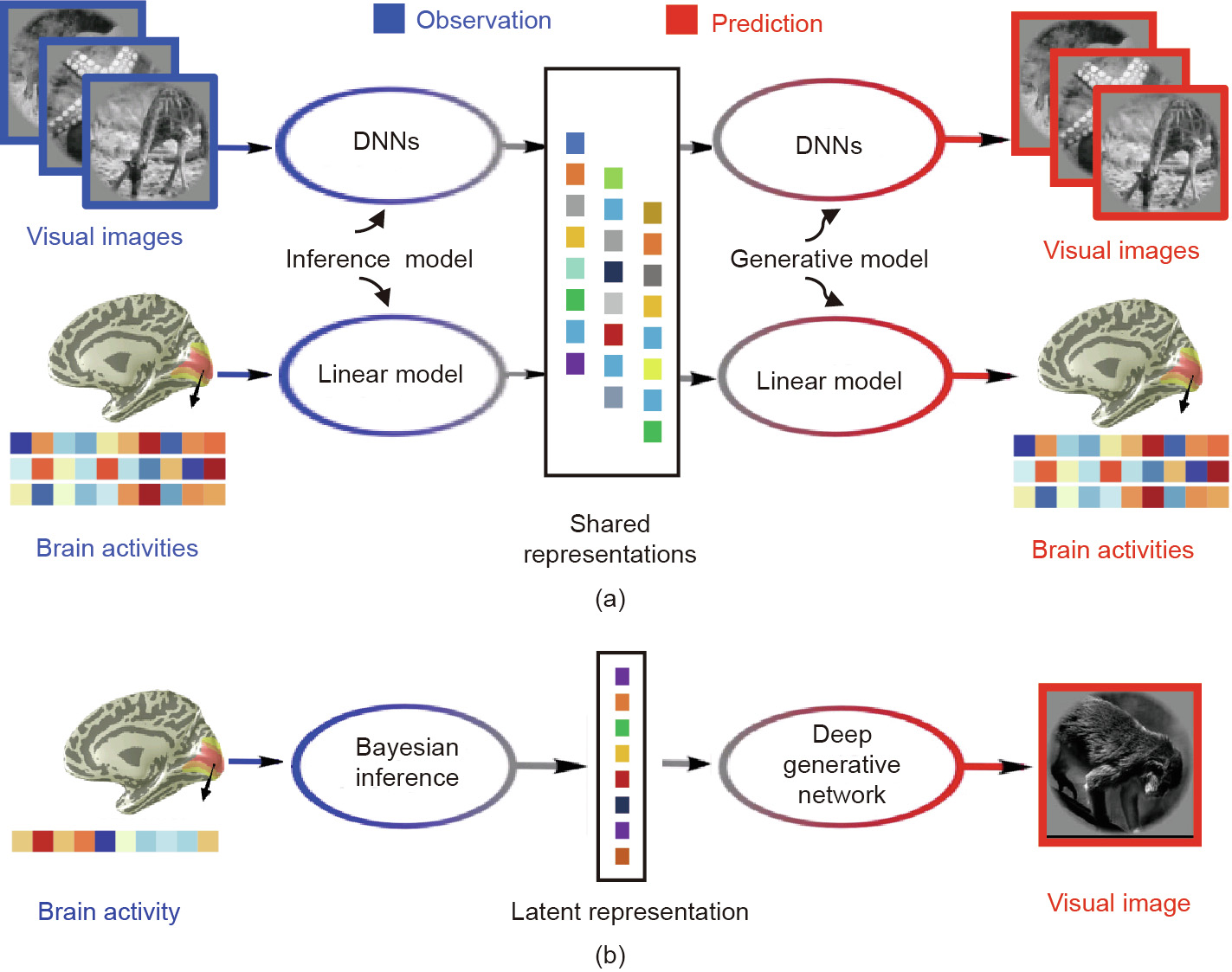
Brain Encoding And Decoding In Fmri With Bidirectional Deep Generative Recon3dmind is introduced, an innovative task aimed at reconstructing 3d visuals from functional magnetic resonance imaging (fmri) signals, marking a significant advancement in the fields of cognitive neuroscience and computer vision and proposes mind 3d, a novel and effective three stage framework specifically designed to decode the brain's 3d visual information from fmri signals. Abstract. brain encoding and decoding via functional magnetic resonance imaging (fmri) are two important aspects of visual perception neuroscience. although previous researchers have made significant advances in brain encoding and decoding models, existing methods still require improvement using advanced machine learning techniques. Furthermore, deep generative models (e.g., variational autoencoders (vaes) and generative adversarial networks (gans)) have produced promising results in studies on brain encoding and decoding. finally, we propose that the dual learning method, which was originally designed for machine translation tasks, could help to improve the performance of. We show that encoding and decoding operations can both be used to investigate some of the most common questions about how information is represented in the brain. however, focusing on encoding models offers two important advantages over decoding. first, an encoding model can in principle provide a complete functional description of a region of.
Comments are closed.