Brain Decoding With Generative Models
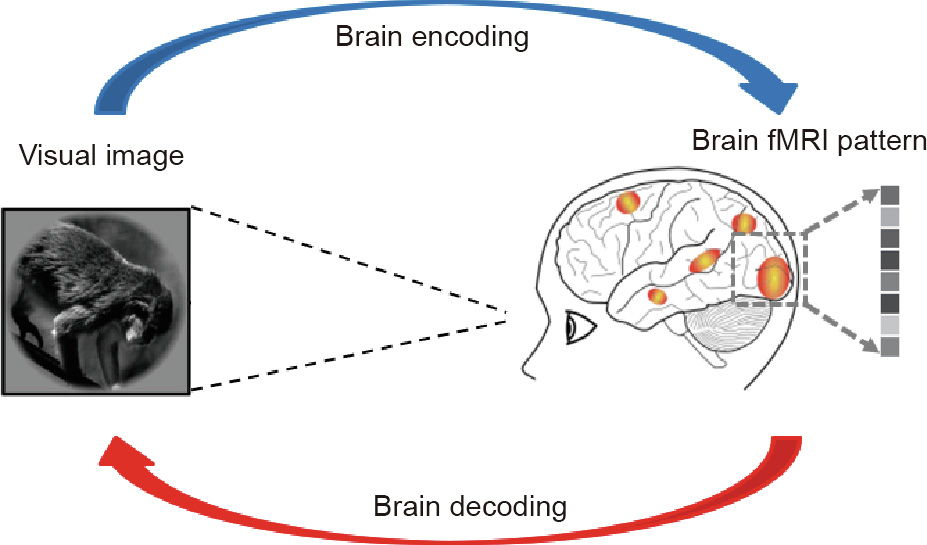
Brain Encoding And Decoding In Fmri With Bidirectional Deep Generative Given the explosion of deep generative models in machine learning and computer vision over the last few years 31,32, the successful application of these methods to brain decoding seemed only a. Abstract. in the past five years, the use of generative and foundational ai systems has greatly improved the decoding of brain activity. visual perception, in particular, can now be decoded from functional magnetic resonance imaging (fmri) with remarkable fidelity. this neuroimaging technique, however, suffers from a limited temporal resolution.
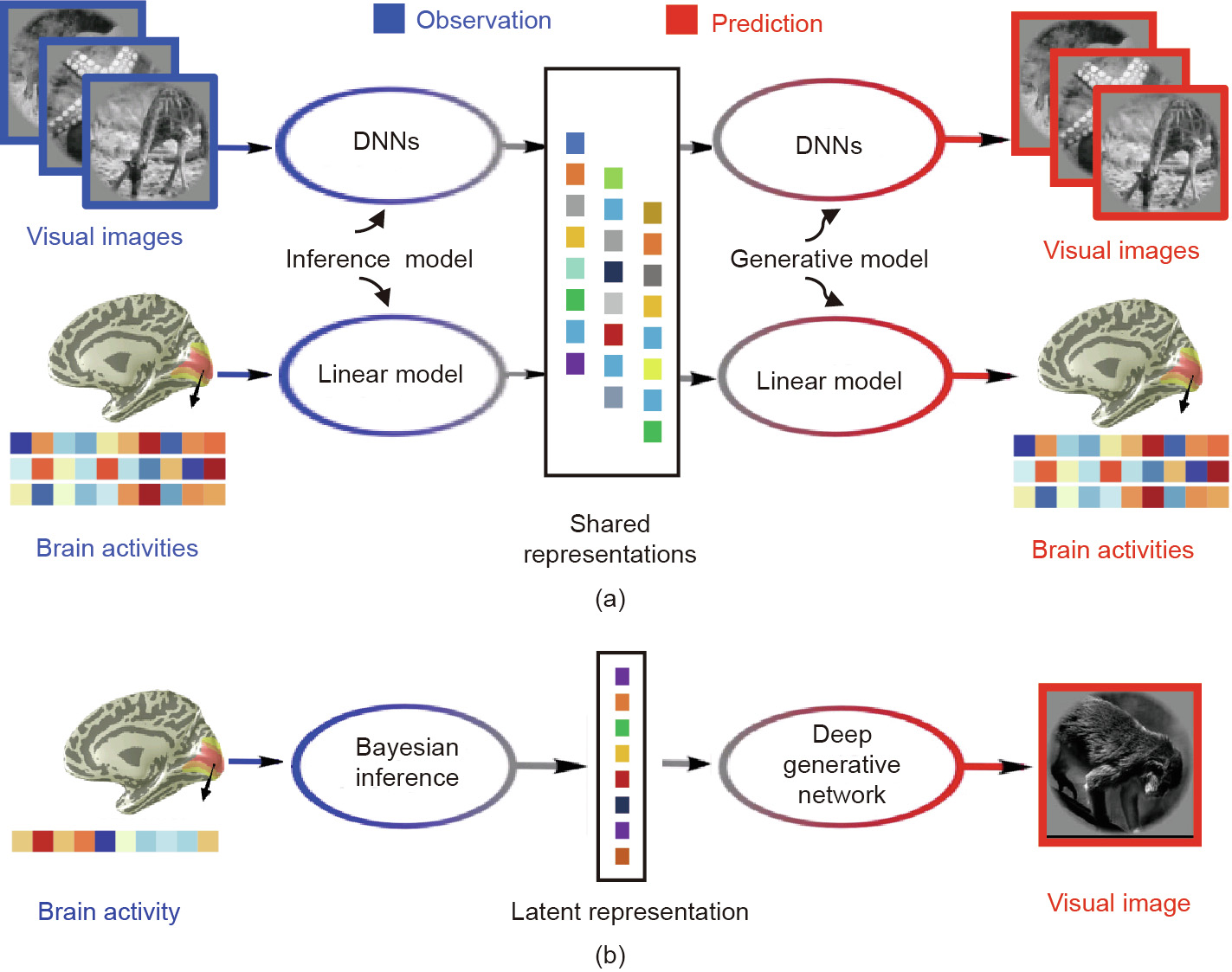
Brain Encoding And Decoding In Fmri With Bidirectional Deep Generative Generative models have become a popular research focus in the field of brain decoding tasks, especially in the reconstruction of perceived images from fmri signals. baek et al. ( 2021 ) proposed a hierarchical deep neural network model of the ventral visual stream to explain the innate emergence of face selectivity. Furthermore, vae based brain decoding models can be found in [50,51,67]. du et al. proposed a structured deep generative neural decoding model, which consists of a structured multiple output regression model (smr) and an introspective conditional generation (icg) model, to reconstruct visual images from brain activity, as shown in figure 2. How to decode human vision through neural signals has attracted a long standing interest in neuroscience and machine learning. modern contrastive learning and generative models improved the performance of fmri based visual decoding and reconstruction. however, the high cost and low temporal resolution of fmri limit their applications in brain computer interfaces (bcis), prompting a high need. Given the remarkable recent success of latent diffusion models 24 in generative ai applications such as text to image generation 24,25,26,27,28, we reasoned that brain decoding studies could also.

Brain Encoding And Decoding In Fmri With Bidirectional Deep Generative How to decode human vision through neural signals has attracted a long standing interest in neuroscience and machine learning. modern contrastive learning and generative models improved the performance of fmri based visual decoding and reconstruction. however, the high cost and low temporal resolution of fmri limit their applications in brain computer interfaces (bcis), prompting a high need. Given the remarkable recent success of latent diffusion models 24 in generative ai applications such as text to image generation 24,25,26,27,28, we reasoned that brain decoding studies could also. At the same time, generative adversarial networks (gans) 16 have emerged as perhaps the most powerful generative models to date 17,18,19 that can potentially bring neural decoding to the next. Unlike almost all brain decoding research that requires training multiple models for different subjects, mindbridge stands out by aiming to achieve cross subject brain decoding with a single model. 2.2. diffusion models diffusion models (dms) [6,8,13,20,36,44,48] have re cently emerged as a focal point in deep generative model re.

Brain Encoding And Decoding In Fmri With Bidirectional Deep Generative At the same time, generative adversarial networks (gans) 16 have emerged as perhaps the most powerful generative models to date 17,18,19 that can potentially bring neural decoding to the next. Unlike almost all brain decoding research that requires training multiple models for different subjects, mindbridge stands out by aiming to achieve cross subject brain decoding with a single model. 2.2. diffusion models diffusion models (dms) [6,8,13,20,36,44,48] have re cently emerged as a focal point in deep generative model re.
Comments are closed.